Google DeepMind can now train small, off-the-shelf robots to compete on soccer fields. In a new paper published today, science robotics, researchers detail their recent efforts to adapt a subset of machine learning known as deep reinforcement learning (deep RL) to teach bipedal robots simplified versions of sports. The researchers note that while similar experiments in the past have created highly agile quadrupedal robots (see Boston Dynamics Spot), far less work has been done on bipedal humanoid machines. He points out that But new footage of the bot dribbling, defending, and shooting on goal shows just how great Coach's deep reinforcement learning can be for humanoid machines.
While Google DeepMind is ultimately aimed at large-scale tasks like climate prediction and materials engineering, it could also completely obliterate human competitors in games like chess, Go, and even the game . StarCraft II. But all these strategic maneuvers don't require complex body movements or coordination. So while DeepMind can study simulated soccer movements, it hasn't been able to translate them to a physical playing field, but that's rapidly changing.
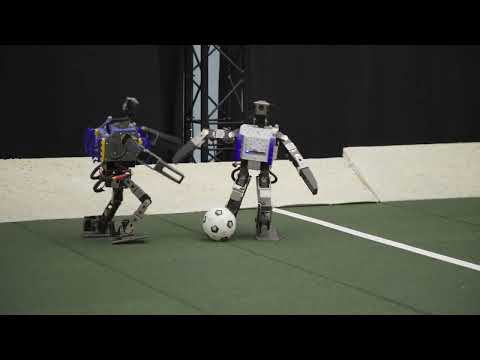
To create a miniature Messi, engineers first developed and trained two deep RL skill sets in computer simulations: the ability to get up off the ground and how to score goals against untrained opponents. From there, they combine these skill sets to virtually train the system to play a full one-on-one soccer match for him, randomly combining them with partially trained copies of themselves. I did.
[Related: Google DeepMind’s AI forecasting is outperforming the ‘gold standard’ model.]
“Thus, in the second stage, the agent combined previously learned skills and honed the overall soccer task, learning to predict and anticipate the actions of its opponents,” the researchers wrote in the paper's introduction. and later states: Agents moved fluidly between all these actions. ”
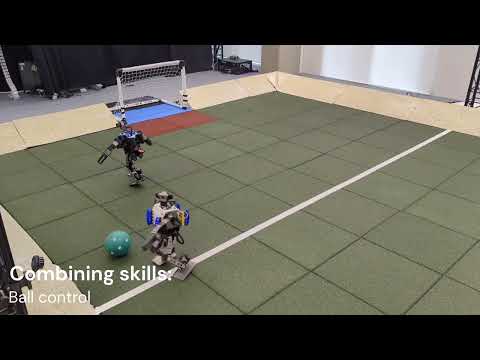
Thanks to the deep RL framework, agents powered by DeepMind can quickly learn how to kick a soccer ball, how to shoot, how to block shots, and even how to defend their own goal from attackers using their bodies as a shield. I learned to improve my abilities.
During a series of one-on-one matches with robots that leverage deep RL training, two mechanical athletes walk and orient faster than if engineers simply provided a scripted baseline of skills. I was able to change, kick, and stand upright. These were no small improvements either. Compared to a non-adaptive scripted baseline, the robot walked 181 percent faster, turned 302 percent faster, kicked 34 percent faster, and stood up from a fall 63 percent faster. Moreover, the robots that underwent deep RL training also exhibited new emergent behaviors, such as turning and rotating with their legs. Otherwise, it would be very difficult to pre-script such actions.
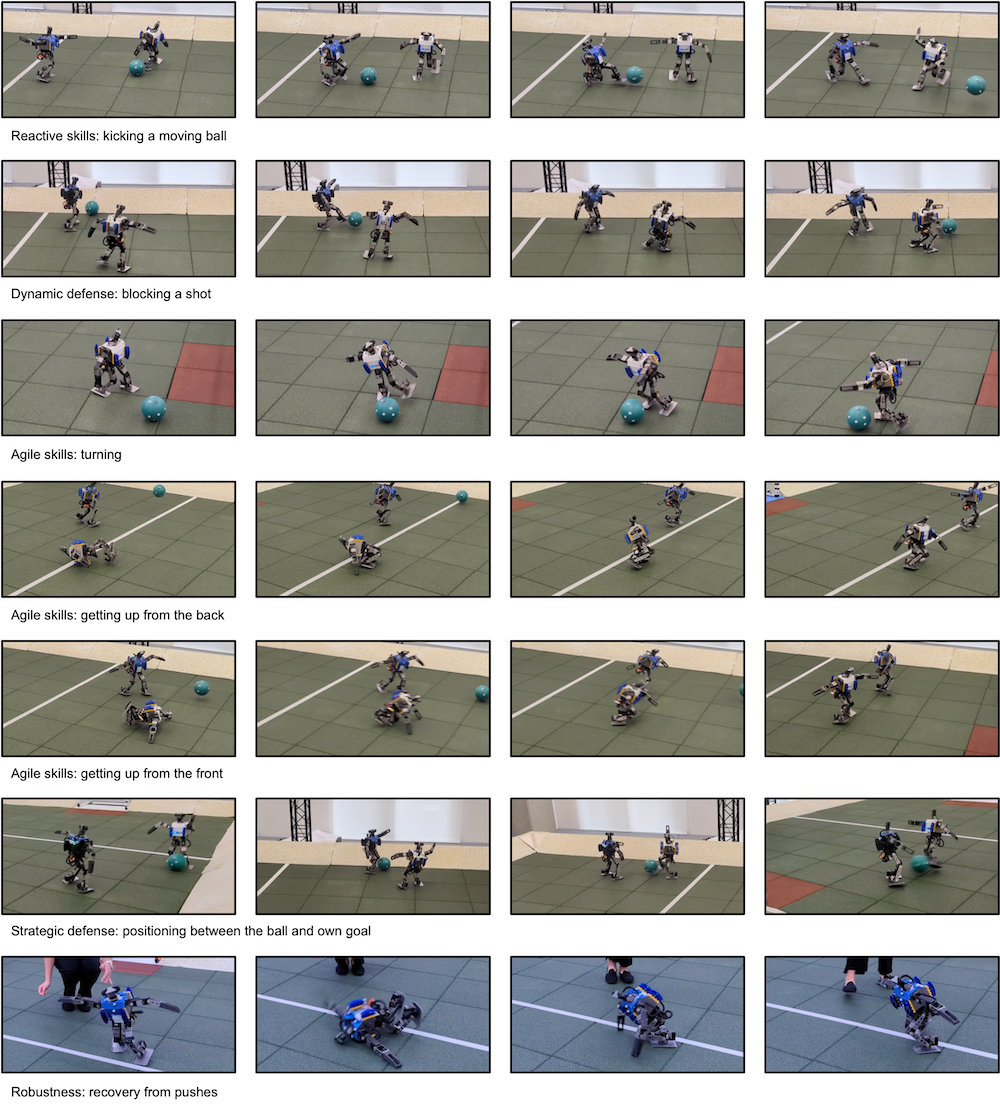
There is still some work to be done before DeepMind-powered robots can compete in RoboCup. In these initial tests, the researchers relied entirely on simulation-based deep RL training before transferring that information to a physical robot. In the future, engineers want to combine both virtual and real-time reinforcement training for bots. They also want to scale up the robot, but that will require a lot more experimentation and fine-tuning.
The researchers believe that similar deep RL approaches for soccer and many other tasks could further improve the movement and real-time adaptation capabilities of bipedal robots. Still, there doesn't seem to be any need to worry about DeepMind's humanoid robots on full-size soccer fields or in the labor market just yet. At the same time, it's probably not a bad idea to be prepared to blow the whistle on them given their continued improvement.